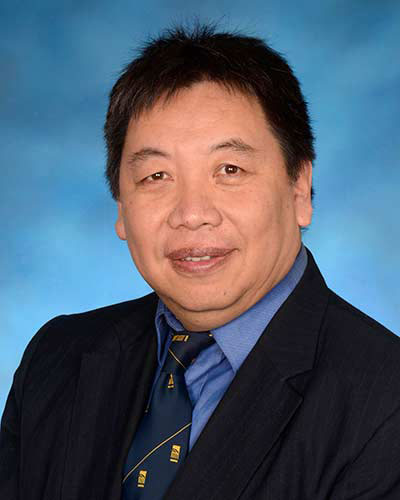
Peter Hu, MD
Faculty Profile
Overview
The research group is composed of research faculty Peter Rock, Professor and Chairman of Anesthesiology; Peter Hu, Associate Professor; Shiming Yang, Assistant Professor, Colin Mackenzie, Professor Emeritus, and Samuel Galvagno, Associate Professor; 2-4 medical students and residents; and 4-8 PhD students in computer science and engineering. Our research is focused on developing machine learning based predictive algorithms for near and long-term patient outcomes based on the continuous analysis of vital signs from the field to in-hospital resuscitation to the intensive care unit bedside.
Our research has been continuously funded by DoD (USAF, Naval Research, US Army and Veterans Administration); past funding agencies included NIH/NLM, NSF, NASA, AHRQ, and industry. Currently, we have over 10 extramural funded projects with over $6 million in funding. We have developed and tested a Bleeding Risk Index (BRI) Monitor for a minute-by-minute analysis of continuous photoplethysmograph (PPG) waveform. This monitor could be used for predicting future transfusion need in the field.
We have also developed an ICU Viewer, which takes real-time patient monitor data and provides an at-a-glance view for thepatients in the SICU, NTCC, MTCC or for an individual patient for up to 7 days.
Meet the Team
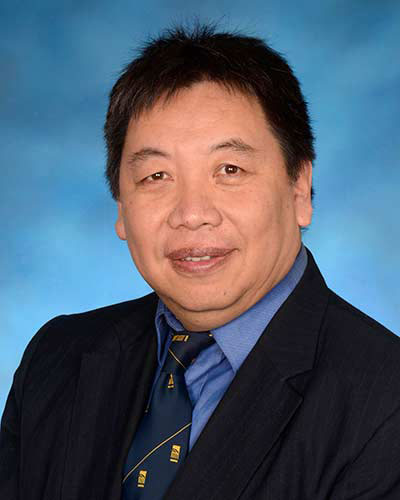
Peter Hu, MS,PhD
Co-Director/ Professor
MS: University of Maryland Baltimore County, USA
PhD: University of Maryland Baltimore County, USA
Interests: Hemorrhagic Shock, Traumatic Brain Injury (TBI), Big Data Analytics, Machin Learning, Real-time patient status estimation and outcome prediction.
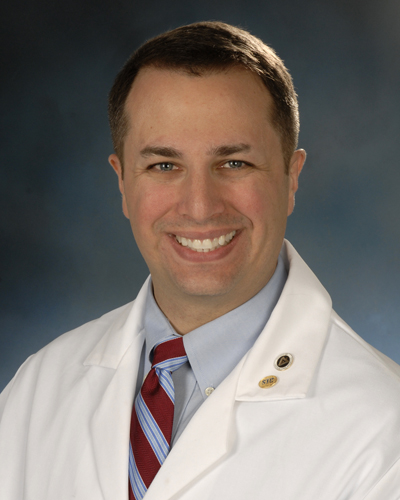
Samuel M. Galvagno, DO, PhD
Professor
Executive Vice Chair of Anesthesiology
Interim Chair of the Department of Anesthesiology
BS (cum laude): Mount Saint Mary's University
DO: New York College of Osteopathic Medicine
PhD: Johns Hopkins Bloomberg School of Public Health
Interests: Aeromedical Critical Care, Patient Monitoring, Resuscitation, Safety & Quality Improvement in the Intensive Care Unit, Medical Education
Colin F. Mackenzie, MB, ChB, MD(res)
Professor Emeritus
Past Director, National Study Center for Trauma
MD: Aberdeen University and School of Medicine, Aberdeen, Scotland
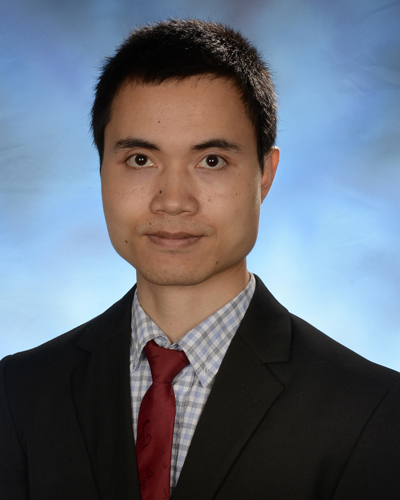
Shiming Yang, PhD
Associate Professor
BS: Applied Math, University of Electronic Science and Technology of China
MS: Applied Math, University of Maryland, Baltimore County (UMBC)
PhD: Computer Science, University of Maryland, Baltimore County
Interests: Large scale data analysis. Medical sensor signal processing. Developing efficient machine learning algorithms to predict life saving interventions and long term outcomes for trauma patients.
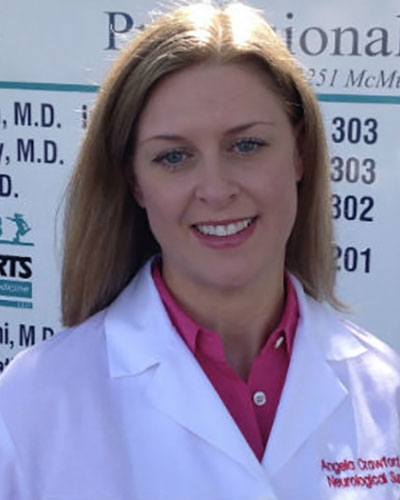
Angela Crawford, MD
Surgery Resident
MD: University of Science, Arts & Technology (USAT), Montserrat, BVI
Resident: University of Maryland, Baltimore, USA
Interests: Trauma Research, Traumatic Brain Injury, Biomarkers, Critical Care, Resuscitative Endovascular Balloon Occlusion of the Aorta (REBOA), Vascular trauma
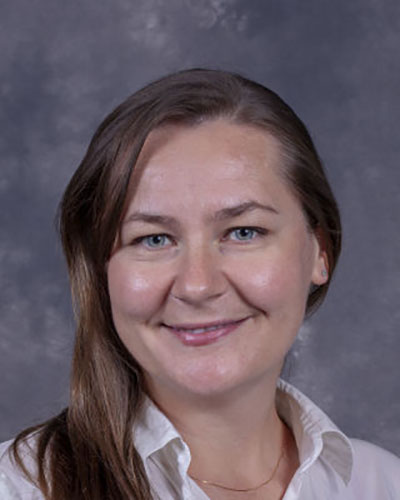
Marta Madurska, MD
Clinical Research Fellow
MD: Jagiellonian Univeristy, Krakow, Poland
MSc: Glasgow University, Glasgow, United Kingdom
Resident: North- East England, UK
Interests: Trauma Research, Vascular Trauma, Endovascular Resuscitation
Additional Members
Thomas Karathanos
ORISE Research Fellow
Chia Chien Lung
PhD Student
Chiene Yu Lin
PhD Student
Rajat Patel
ME Student
YI Mei Kuo
PhD Student
Daniel Curtis
Programmer
Rajan Patel
Programmer
Serenity Miller
Research Assistant
Projects
Oximetry and Non-invasive Predictors Of Intervention Need after Trauma
Six projects (ONPOINT 1-6) separately funded by Department of Defense are covered by the ONPOINT umbrella.
Figure 1: Patient care pathway in Maryland EMS and Shock Trauma Center. A, B, and C are the phases of care (prehospital, initial evaluation, and resuscitation, respectively) where subject VS data has been collected for the ONPOINT 1-6 efforts.
ONPOINT 1 used pulse oximetry photoplethymographic (PPG) signals to predict which trauma patients would be given blood within 1, 6, 12 or 24 hours of Shock Trauma Center (STC)admission using the first 15 minutes of PPG waveforms collected after STC arrival, and using historic pre-hospital data.
ONPOINT 2. Continuous pulse oximetry and ECG waveforms were recorded for direct-admit trauma patients with abnormal pre-hospital shock index ≥ 0.62 (N = 1200). SBP recorded every 2 seconds from NIBP cuff n=1200 Outcomes using PPG and ECG features. Tested algorithm predictions of transfusion with Receiver Operating Curves (ROC) for Blood transfusion 1-3hr, 1-6 hr, 1-12hr: massive transfusion, 5 Units within 4 h (MT1), ≥10 units within 12 (MT2), 24 h (MT3) (Table 1).
Table 1:
ONPOINT 3: Continuous physiologic patient data from electrocardiographic (ECG) and photoplethysmographic pulse oximetry waveforms (PPG) were captured from the first contact with a casualty in the field through transport and handoff to advanced care. The decision-assist algorithms recognize critical hemodynamic changes as they are happening, update this information in a clinically timely and useful format, and are used to alert clinicians to the potential need for transfusion in a valid, reliable, and entirely automated fashion. This work is an immediate proximate step toward translation into analytic platforms and prototype hardware for deployable instrumentation for the field and hospital use.
ONPOINT 4: Emergency blood transfusion prediction algorithms based on the civilian trauma patient population demographics may not be useful to predict hemorrhagic shock in a military population. ONPOINT 4 stratified sampling to select a subset of civilian patients who were similar demographically to the military population in terms of age, injury severity, the rate of transfusion, and gender. We hypothesized that an algorithm for predicting blood transfusion based on these stratified data from 22,819 adults (age ≥18 y) civilian trauma patients can accurately predict the need for transfusion in a military population. The blood transfusion prediction algorithm predicted uncross-matched (UnX) blood and massive transfusion (MT) with AUROCs of 0.82 and 0.90 respectively. A subset of 1200 patients was developed with characteristics of military population, e.g. age<56, 85% male, high rate of penetrating injuries (33%), and high transfusion rate (25%). The same algorithm was evaluated using this subset of patients with prediction results of AUROCs of 0.85 UnX and 0.91 MT. All results were statistically significant (p<0.001). The model performed better than other available MT prediction models (Figure)
ONPOINT 5: Our previously developed prediction algorithms were adapted for use with patient VS of patients collected continuously during transport from the scene of injury via helicopter. The resulting optimization will provide enhanced predictions on which patients should receive uncrossmatched blood type O blood (universal donor [UnxRBC]), massive transfusion (>5 units of blood within 4 hours of admission [MT]), emergency life-saving surgery within 24 hours admission, and ICU admission of three or more days duration. See Table 2 and Fig 2 below
Table 2 Predictions of transfusion (PRBC) at 1- 3 hours, and Massive Transfusion (MT) by Area Under Receiver Operating Curve (AUROC)
Figure 2: ROC curves for the four scoring systems / models for predicting MT, where RTS= Revised Trauma Score SI= Shock Index, BRI = Blleding RiskIndex (The algorithms developed by ONPOINT) and ABC= Assessment of Blood Consumption.
ONPOINT 6: Our hypothesis in ONPOINT 6 is that a validated Decision-Assist From All Available Sources (DAFAAS) algorithm, using electronic data streams that are readily available at all echelons of military and civilian casualty care, from field assessment through transport and trauma center admission, can accurately and reliably predict the need for transfusion and provide a surrogate marker for uncontrolled bleeding and the need for life-saving-interventions (LSIs) and trauma team readiness. Our overall objectives in demonstrating this hypothesis are 1) to refine, test, and validate the available Maryland Shock Trauma (STC) Center DAFAAS algorithm in large cohorts of retrospective (more than 10,000 trauma patients), perspective (more than 1,000 trauma patients), trauma center and pre-hospital data gleaned from military-appropriate physiological monitoring systems, and 2) to confirm that the DAFAAS algorithm meets the same transfusion prediction specification after military-compliant-compatible wireless transmission. Our overall goal is to develop a reliable decision-support tool that can accurately distinguish those who need early or large scale transfusion from those who don’t, to save a life, minimize complications, and reduce unnecessary blood use in civilian and military populations. This project is on-going and application is underway to FDA for 510K approval of the DAFAAS algorithm. An overview of ONPOINT 6 is given in Figure 3 below.
Figure 3:
Critical Care Air Transport Team (CCATT) Viewer for Advisory Alarms, Situational Awareness, Mentoring and Remote Decision-making
Develop and Test a Critical Care Air Transport Team (CCATT) viewer to allow improved situation awareness while operating a portable intensive care unit (ICU) onboard any military transport aircraft during flight (Figure 5). Test and evaluate by CCATT subject matter experts, both the hard wired and ruggedized wireless CCAT Viewer system using existing real vital signs data for brain-injured patients (intra-cranial and cerebral perfusion pressure, Brain Trauma and Shock Index) who required Brain Trauma Foundation recommended interventions to determine if the centralized CCATT Viewer enhances earlier recognition of intervention need.
Figure 5:
Developing Reliable Telemedicine Platforms with Unreliable and Limited Communication Bandwidth
Telemedicine techniques could deliver health care services to battlefields and expedite and optimize patient care and triage. However, the communication networks in such environments are often unreliable and have limited bandwidth. In this study, we evaluated the accuracy loss and clinical decision error caused by data transmission.
We compared physiologic data under various resampling rates with the original high-resolution data and reported the matrix to a detailed trade-off between clinical accuracy and band resource requirement. Using data collected from 15,000 trauma patients, we found that heart rate readings could be within a 10% difference when reducing sampling rate from 2 seconds to 5 minutes. Moreover, the high-fidelity waveform could be reduced for sampling rate with a small (<5%) loss in prediction performance.
This study could guide the optimal selection of data sampling rate and estimation of data reliability as well as in the future determine any impact o sampling rate on clinical decisions.
Figure 5: Scatter plot (left) of instantaneous HR sampled every 5 min vs. the median HR calculated from the corresponding windows with bounds as ±10 bps or ±10%; illustration of the density of data points (right).
Assessment of Surgical Performance
Test with an individual procedure score (IPS) whether an unpreserved cadaver trauma training course including upper and lower limb vascular exposure improves correct identification of surgical landmarks, underlying anatomy, and shortens time to vascular control for residents, practicing surgeons and experts Forty 2nd – 6th year surgical residents from 13 surgical programs throughout the Mid-Atlantic States who had not previously taken the ASSET course were enrolled, 40 completed the pre-post –ASSET performance evaluations.
After ASSET training, all components of IPS increased and time shortened for each of the three artery exposures. Procedure steps performed correctly increased by 57%, anatomic knowledge increased 43% and skin incision passage of a vessel loop twice around the correct vessel decreased by a mean of 2.5 minutes. An overall vascular trauma readiness index, a comprehensive IPS score for three procedures increased 28 % with ASSET Training, IPS is a benchmark for competence in extremity vascular control.
Thirty-eight of these same forty residents were evaluated before and within 4 weeks of the ASSET, training completed evaluations 12- 18 months later. Overall resident cohort performance improved (p <0.001) after ASSET regardless of clinical year of training. Comparing post- to pre-training, residents demonstrated increased anatomy knowledge, and correct procedural steps, decreased errors from 60% to 19%, and reduced time to complete vascular procedures. For 21 of 40 residents, correct procedural steps plotted against anatomy knowledge (the two IPS components most improved with training) show resident’s performance was within one nearest-neighbor classifier of experts after ASSET training. Five residents showed no improvement with training. Experts were significantly (p <0.05) better performers compared to trained residents. Critical errors that might lead to patient death were predicted by pre-training decile of IPS < 0.5. At follow-up, the frequency of critical errors was no different from the experts. Performance varied nearly 100% among residents for core trauma competency procedures. Interval experience, rather than time since training, impacted skill up to 18 months later. Sixty percent of experts and residents failed to decompress or confirm entry into all fasciotomy compartments.
Residents, practicing and expert surgeons were evaluated mean 14months (38 residents), 30 months (35 practicing surgeons) and 46 months (10 experts) after ASSET training. Practicing surgeons had lower IPS and component scores than residents and experts and made more critical errors than residents or experts.
Cluster analysis (Figure 8) of anatomy vs procedural steps identified tertiles of performance and wide variance (32.5 % practicing surgeons, 26.5%residenst, and 13% experts). Vascular control duration > 20 minutes (n=21) and failure to decompress lower extremity fasciotomy were correlated with incorrect landmarks and skin incision placement. Practicing surgeons with low trauma skills experience since training had a lower individual procedure and component scores and more errors compared with experts and residents. Surgeons, including experts with low interval experience performing trauma procedures, may benefit from refreshing of correct landmark and skin incision placement identification.
Figure 8: Cluster Analysis of correct procedural anatomy skills and technical steps by resident (blue = pre- training, red = same residents post-training ) and expert surgeons (green). Circles represent one-nearest neighbor form the centroid of each cohort. JAMA Surg. 2017 Jun 1;152(6):1-8.
Figure 9: Cluster Analysis of correct procedural anatomy skills and technical steps by resident (red), practicing surgeons (blue) and expert surgeons (green). One standard deviation (SD) of each nearest neighbor around their centroid is shown by colored circles. (J Am Coll Surg 2018. In Press)
Figure 10: Critical Technical and Morbidity Errors and Error Recovery per surgeon among resident, practicing and expert surgeon cohorts (J Am Coll Surg 2018. In Press)
Grants
Grant Information | Dates |
---|---|
Joint Warfighter Medical Research Program (JWMRP) W81XWH-17-C-0034 Predicting Blood Transfusion Using Automated Analysis of Vital Sign Input From All Available Sources PI: Thomas Scalea MD. ISR PI: Jose Salinas, PhD ; TATRC PI: Gary Gilbert, PhD Role: Main PI |
2017 - 2020 |
DoD: Discovery Program FA8650-17-2-6H11 Emergency Preservation and Resuscitation: Novel Application of ECMO and Closed-Loop Physiologic Monitoring USAFSAM_FHE PI: Catriona Miller, PhD; OHSU PI: James Rose, PhD Role: Main PI |
2017 - 2020 |
FA8650-17-2-6H09 United State Air Force Pre-Hospital Automated Prediction of Blood Product Needs After Severe Trauma: A Prospective Study USAF PI: Dr. Stacy Shackelford, Col, USAF, MC; Catriona Miller, PhD Role: PI |
2017 - 2020 |
USAF Contract FA8650-18-2-6H16 Optimal Vital Signs Processing Methods for Telemedicine Application in Limited Bandwidth Environments (Telemed II) PI: Shiming Yang, PhD Role: Co-PI |
2018 - 2019 |
USAF Contract FA8650-18-2-6H18 Real Time Vital Sign Assessment to Predict Neurological Decline After Traumatic Brain Injury (RAPID-TBI) PI: Neeraj Badjatia MD. Co-PI: Shiming Yang PhD Role: Co-PI |
2018 - 2021 |
United States Air Force Contract FA8650-15-2-6D24 Assessing the Accuracy and the Impact of Standard-Practice Ventricular Drainage on Intracranial Pressure Measurements following Traumatic Brain Injury” PI: Deborah Stein, MD Co-PI: Bizhan Aarabi, MD Role: Co-PI |
2016 - 2019 |
United States Air Force Contract: FA8650-16-2-6H05 Evaluation of Handoff Outcomes in a Multi-Site Continuum of Care Exercise (HandOff) PI: Thomas Grissom Role: Co-PI |
2015 - 2018 |
Publications
Please see Dr. Hu's faculty profile for a complete list of his publications.
You can also see his publications on PubMed.
Contact
Office: 410-328-7264
Email: phu@som.umaryland.edu